Shen Liang
June 15, 4 PM
online (zoom)
Abstract
This tutorial provides an overview of two important and correlated (in many cases intersectional) topics in deep learning: transfer learning, and multi-task learning. Transfer learning focuses on transferring knowledge learned in one problem to a different yet related problem, while multi-task learning attempts to solve multiple tasks in one stroke by exploiting the commonalities across the multiple tasks. In this tutorial, I will present an overview of the motivations, methodologies, as well as applications of these two learning paradigms in fields such as natural language processing and computer vision. I will also provide two concrete examples of these paradigms in a hands-on workshop.
Dr Shen Liang
(Université Paris Cité, diiP)
Shen Liang is a research associate at the Data Intelligence Institute of Paris (diiP) and affiliated with the Université Paris Cité. He has worked on a variety of data management and mining problems including time series analysis, semi-supervised learning, knowledge-guided deep learning and GPU-accelerated computation within various fields such as healthcare, manufacturing, geosciences and astrophysics. He holds a PhD in software engineering from Fudan University, China.
Other seminars
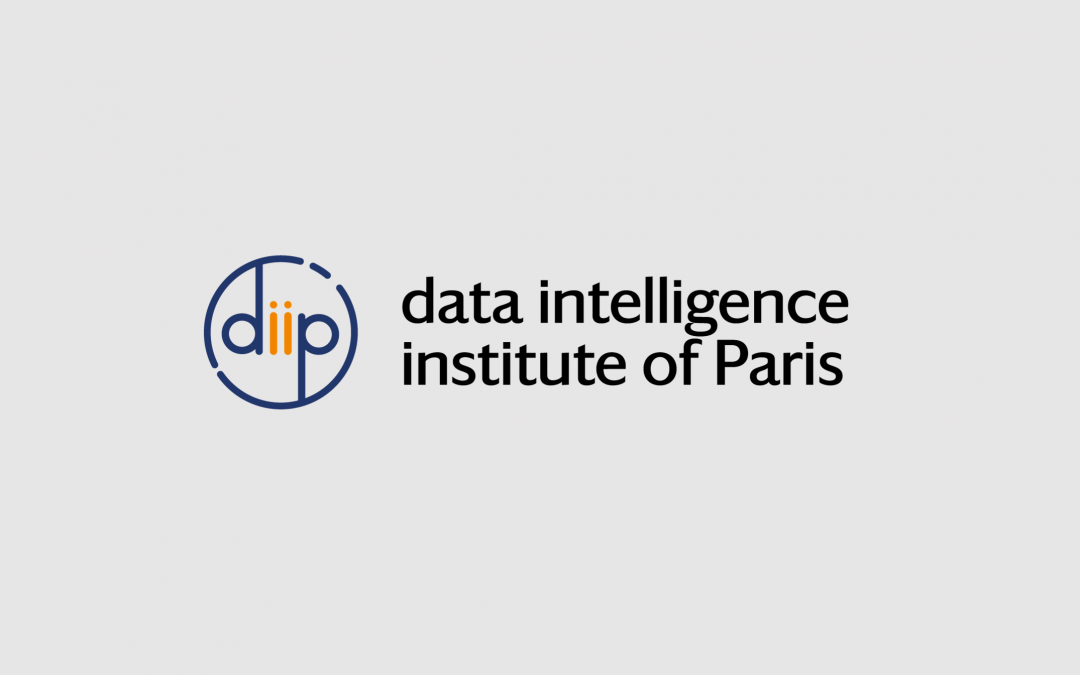
Shen Liang – Knowledge-guided Data Science
Shen LiangMay 18, 4 PM online (zoom) linkedinAbstract This tutorial presents an overview of knowledge-guided data science, a rising methodology in machine learning which fuses data with domain knowledge. We will present numerous case studies on this methodology...
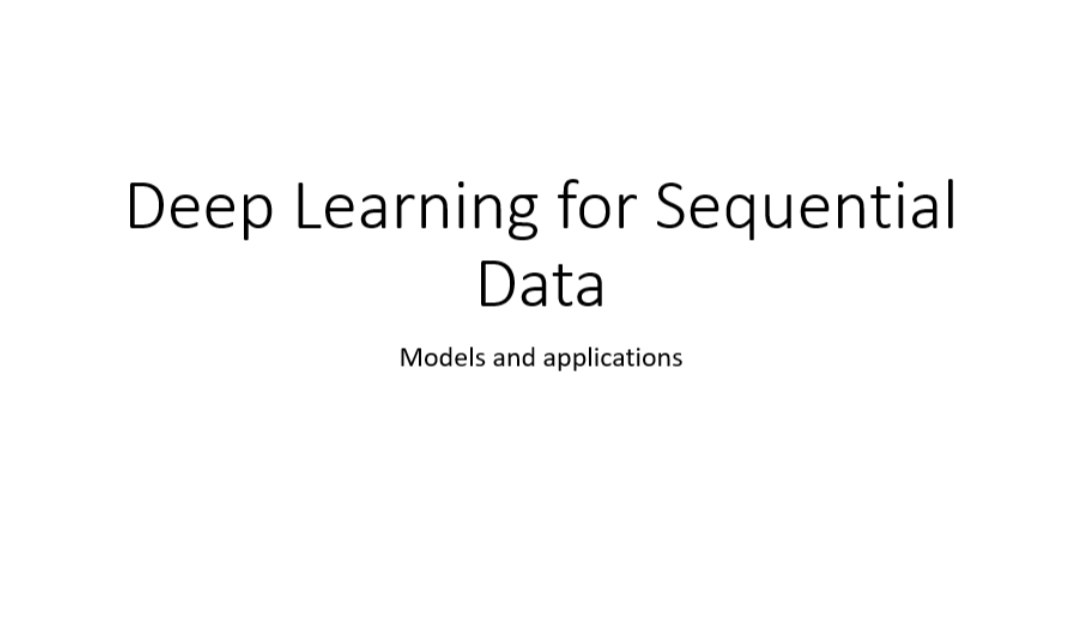
Foula Vagena – Deep Learning for Sequential Data: Models and Applications
Foula VagenaApril 13, 4 PMonline (zoom) Abstract Recurrent neural networks (RNNs) are a family of specialized neural networks for processing sequential data. They can scale to much longer sequences than would be practical for networks without sequence-based...
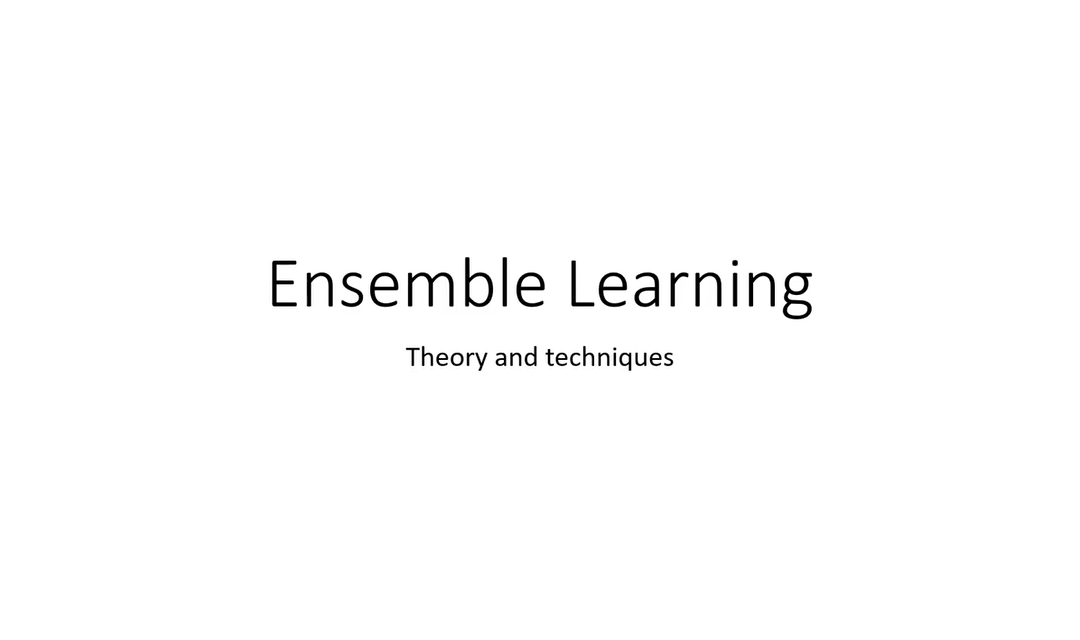
Foula Vagena – Ensemble Learning: Theory and Techniques
Foula VagenaDecember 15, 4pmonline (zoom) Abstract Ensemble learning is the process by which multiple models, such as classifiers or experts, are combined to solve a particular computational intelligence problem. Ensemble learning is primarily used to improve...
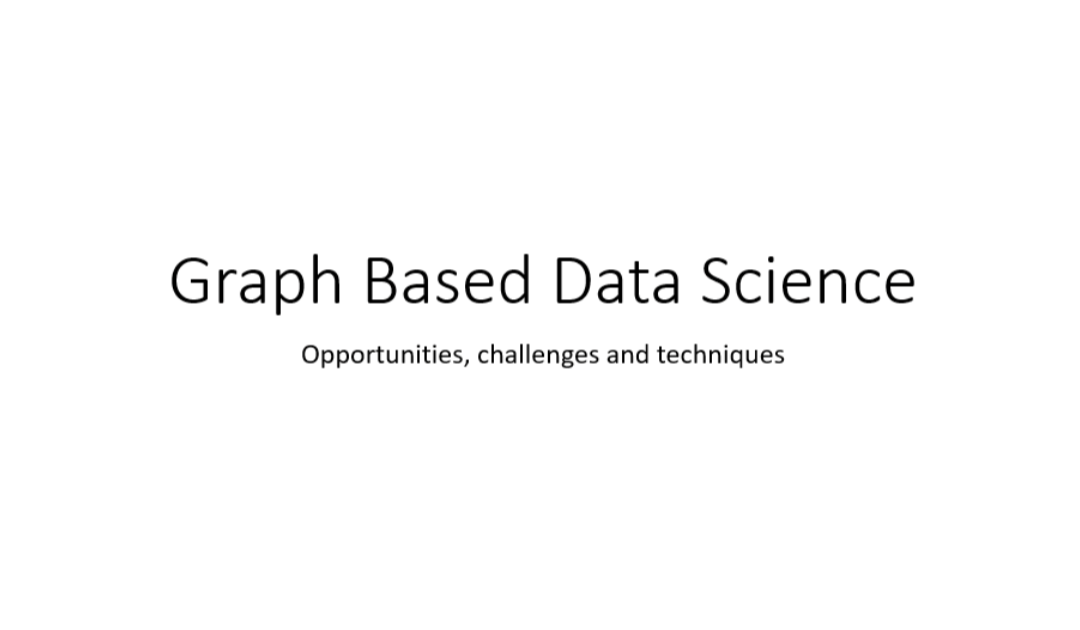
Foula Vagena – Graph Based Data Science: Opportunities Challenges and Techniques
Foula VagenaJanuary 19, 4pmonline (zoom) Abstract Graph based data science lets us leverage the power of relationships and structure in data to improve model prediction and answer previously intractable questions. In this tutorial we will first introduce the...