2024
Strategic Projects
@Physics/Astronomy
#Self-supervised Learning
#Semi-supervised Learning
#Sensitivity enhancement
#Data Analysis based on AI
Project Summary
The aim of the ADAPT project was to develop a novel analysis strategy for the KM3NeT astroparticle physics experiment, using semi-supervised learning, to minimise the dependence on labelled data coming from Monte Carlo simulations. KM3NeT, a neutrino telescope located deep in the Mediterranean Sea, consists of a 3D array of Cherenkov light detectors that capture the passage of charged particles induced by neutrino interactions. A key challenge in KM3NeT data analysis is the substantial light background caused by K40 decays in seawater, which surrounds each neutrino-induced event. We will present our results on the implementation of this innovative analysis strategy.
Yvonne Becherini
yvonne.becherini@u-paris.fr
- Professor at Université Paris Cité
Ankur Sharma
Pranju Goswami
Maximilian Eff
Enzo Oukacha
Projects in the same discipline
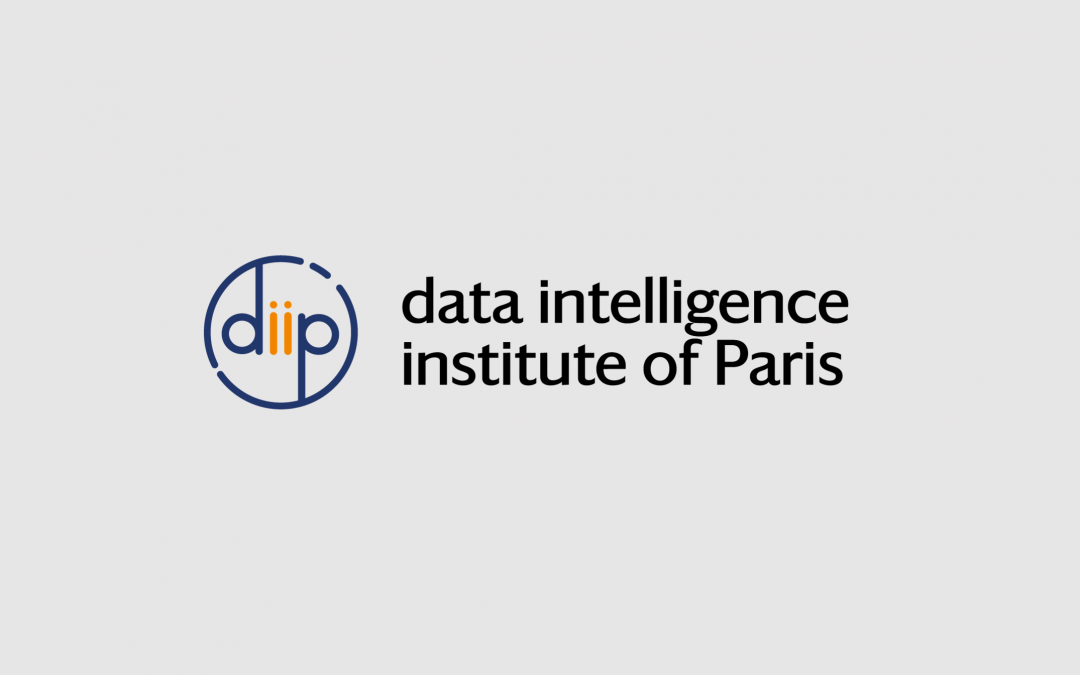
Deeply Learning from Neutrino Interactions with the KM3NeT neutrino telescope
2022 PhD/ DIAI Projects @AstronomyParticle physics and graph neural networks Santiago PENA MARTINEZ Project Summary A new generation of neutrino experiments is in the horizon looking to explore many of the open questions on neutrino properties and searching for...
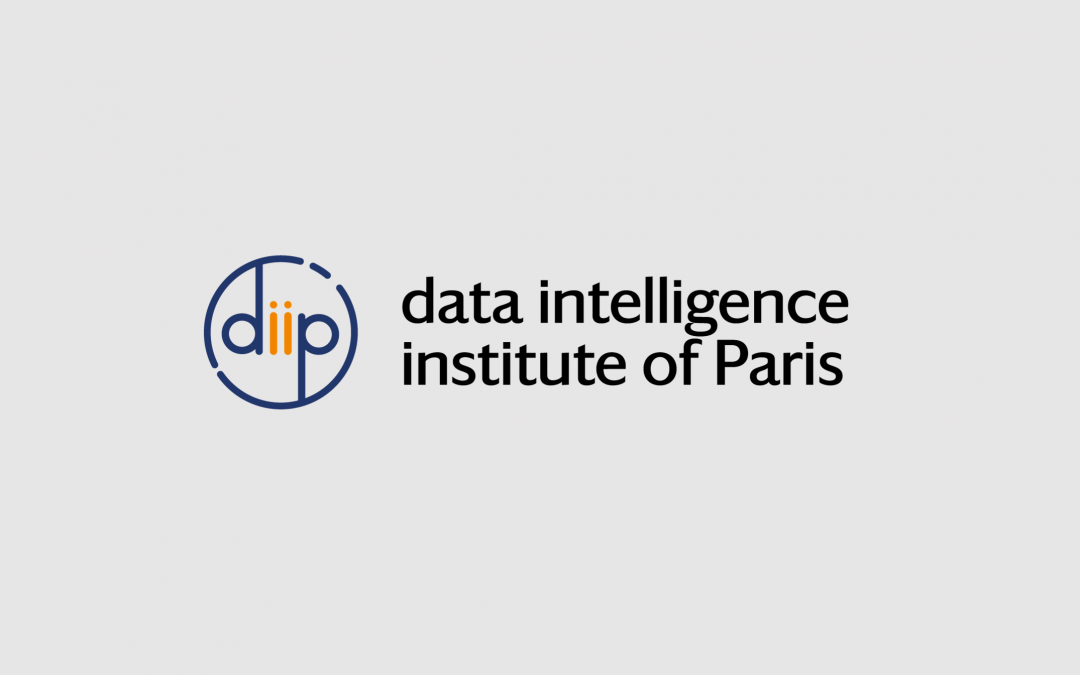
Learning the magneto-ionic side of the turbulence in the interstellar medium in radio-astronomy
2022 PhD/DIAI Projects @AstrophysiqueJack Berat(LPENS, UPC)Project Summary The phase transition from warm neutral medium to cold neutral medium in the interstellar medium (ISM) is affected by the magnetic field. The polarization of the synchrotron emission is one of...
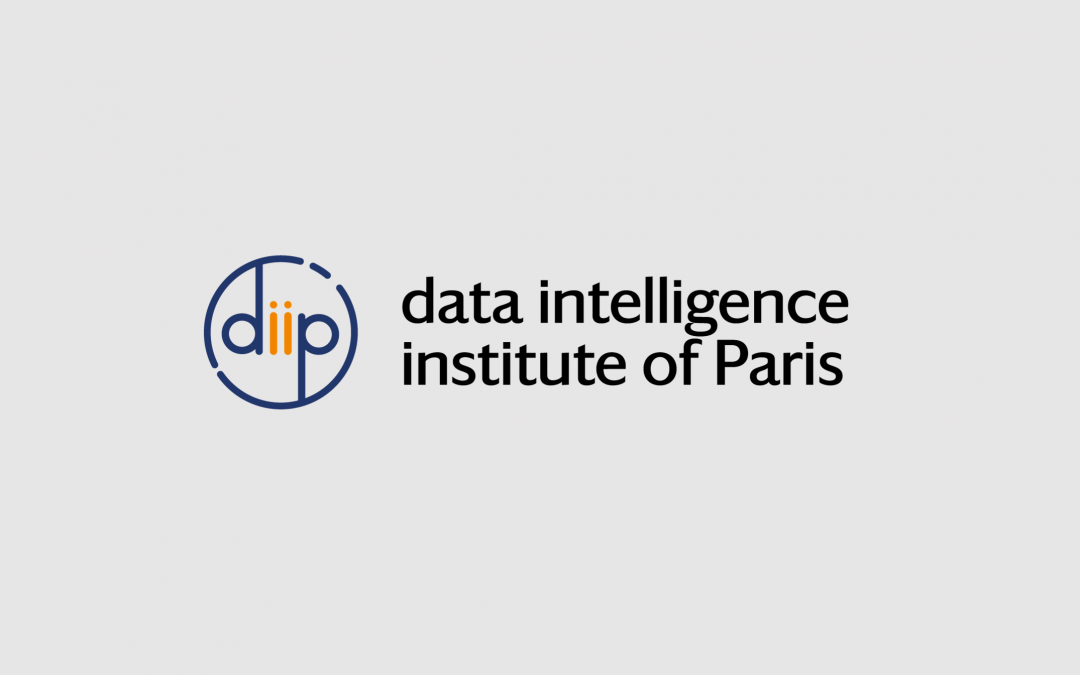
Machine Learning for Photometric redshift estimation of LSST galaxies
2023Masters Projects@Physics & Astronomy +Computer Science+Mathematics/Statistics #machine learning#convolutional networks#astrophysics Project Summaryto be updated. Simona Mei Projects in the same discipline
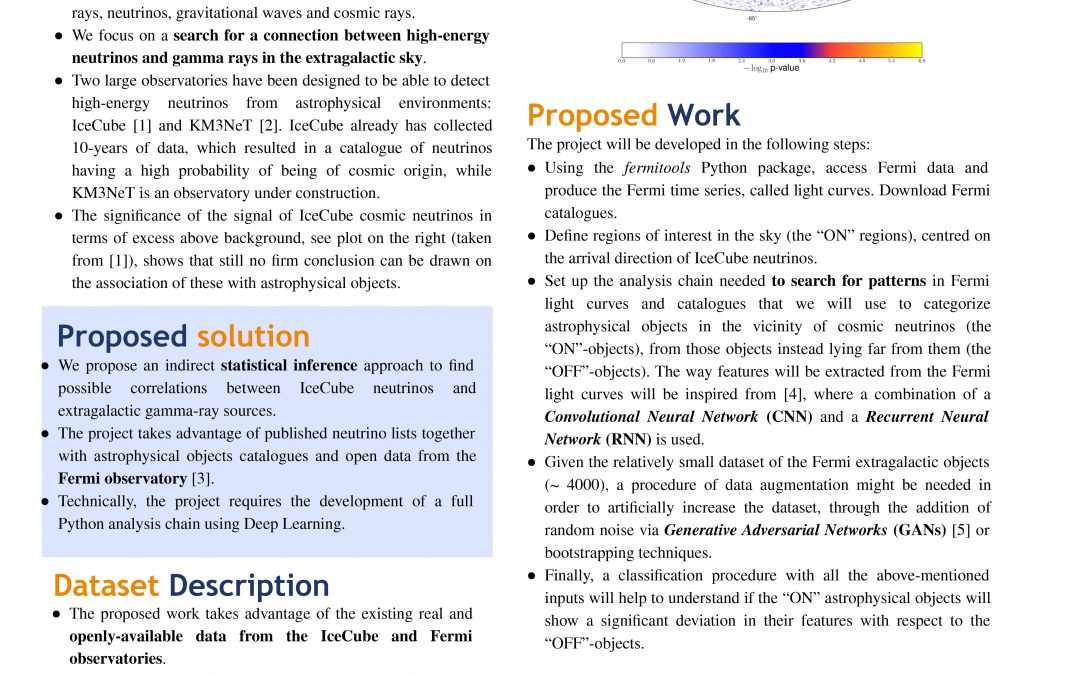
“Search for features in astrophysical objects close to cosmic neutrinos”. An indirect approach to cosmic neutrino association with astrophysical objects
2022Masters Projects@Physics & Astronomy +Computer Science #Neutrino and Gamma-Ray Astronomy#Deep Learning#Real Data Analysis#Data Augmentation Project SummaryThe work proposed here is in the field of Astroparticle Physics, a sub-branch of Physics dealing with the...