2024
Strategic Projects
@Computer Science
#Machine Learning
#Data Drift
#Fairness
Project Summary
Machine learning (ML) is increasingly used to make decisions that highly impact applications like hiring, medical diagnosis, education and criminal justice, etc. All of these applications directly impact people’s lives, and can harm our society if not designed and engineered with considerations to fairness and ethics. Potential sources of fairness issues in machine learning predictions, can arise from biases in the data causing unfair decisions. One form of bias is data drift, which refers to changes in the distribution of features (e.g., gender, race, sex, age, and others). It may be harmful, because it can negatively impact the performance of machine learning models in a significant way, leading the models to inaccurate predictions and unfair decision-making.
In this project, we focus on gender fairness caused by drifts. Many existing works study the existence and effects of gender disparities in ML applications, and affirm they are observed in society at various levels and perpetuated in ML applications. For example, in hiring applications, it’s demonstrated that job applicants are affected and related to gender stereotypes, e.g., men tend to apply for more technical and ambitious jobs as compared to women. According to the division of gender equality of UNESCO, ML systems used in the recruitment software are found to be biased towards women. Hence, still more effort and work are required to mitigate gender disparities and make ML applications fairer for all users.
In social science, it is crucial to ensure that social studies accurately reflect the experiences and perspectives of people of all genders. This allows to ensure that data used in ML applications to be representative and their outcomes are not harmful. Gender fairness is then an ongoing effort that requires collaboration between data scientists and social scientists, and other diverse user groups. By considering gender fairness as a fundamental aspect of model performance, ML applications can better serve all users while avoiding to reinforce harmful disparities or stereotypes. For example, in applications using demographic data for recruitment to predict job suitability or promotions, it is important to ensure that the model provides equal opportunities for genders.
Our objective is hence to investigate the social science perspective of gender fairness in ML applications. Our contributions will reflect the collaborative efforts and shared interests of computer science and social science fields. Setting such interdisciplinary research is a challenge in itself, starting from finding a common terminology and definition of fairness, which is not trivial.
Soror Sahri
soror.sahri@parisdescartes.fr
- Associate Professor, UPCité
Philipp Brandt
Assistant professor of sociology – SciencesPo
Marc Hulcelle
Postdoc – diiP, UPCité
Sijie Dong
PhD student – LIPADE, UPCité
Projects in the same discipline
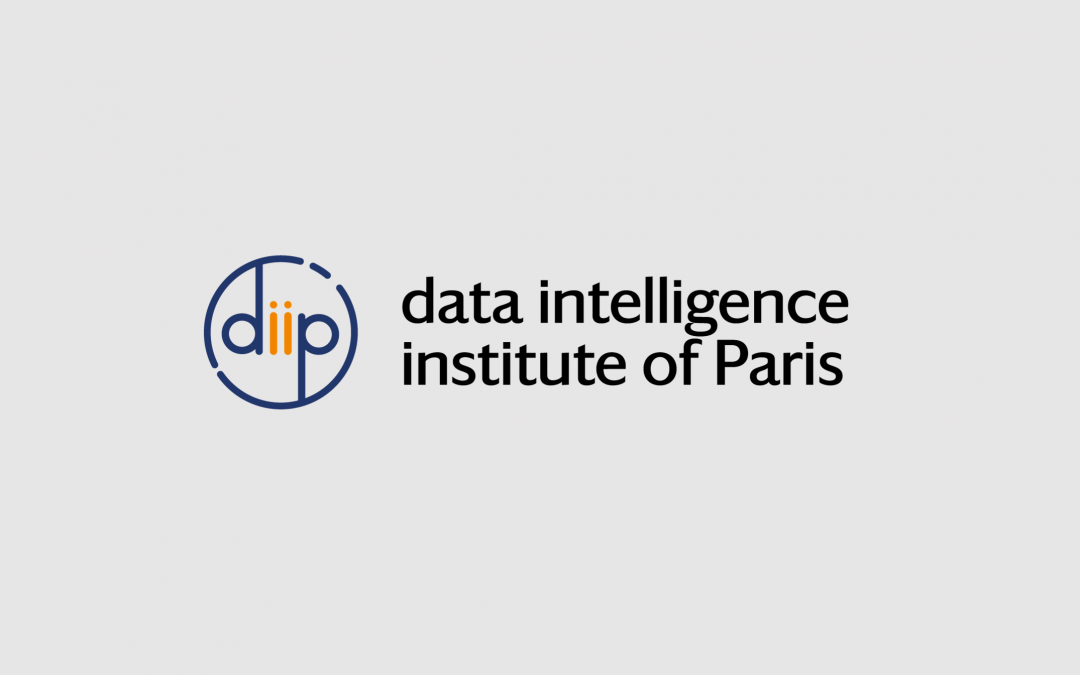
Diffusion Models Based Visual Counterfactual Explanations
2024 Masters Projects @Computer Science #Visual counterfactual explanations #Diffusion Models #Identification of subtle phenotypesProject Summary to be updated Valerie MezgerProjects in the same discipline
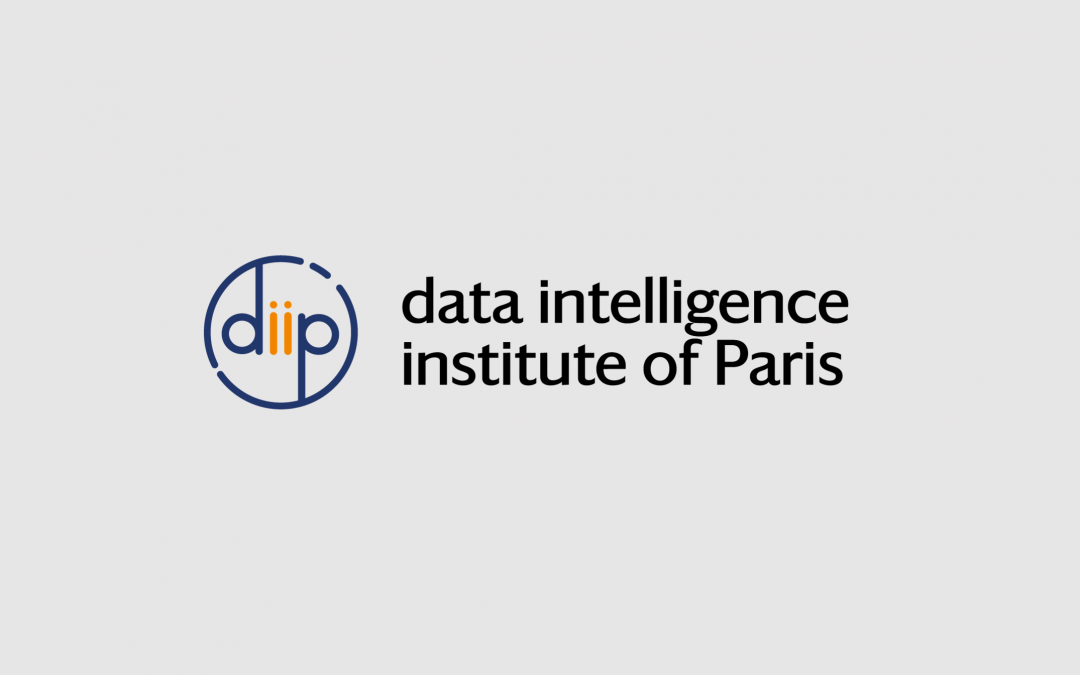
OpenStreetMap and Sentinel-2 data for the production of environmental indices for demographic studies
2023Masters Projects@Computer Science +Demography #Remote sensing#Demography#Deep learning#Sentinel 2#OpenStreetMap#Local climate zones#Africa Project Summaryto be updated. Sylvain Lobry Projects in the same discipline
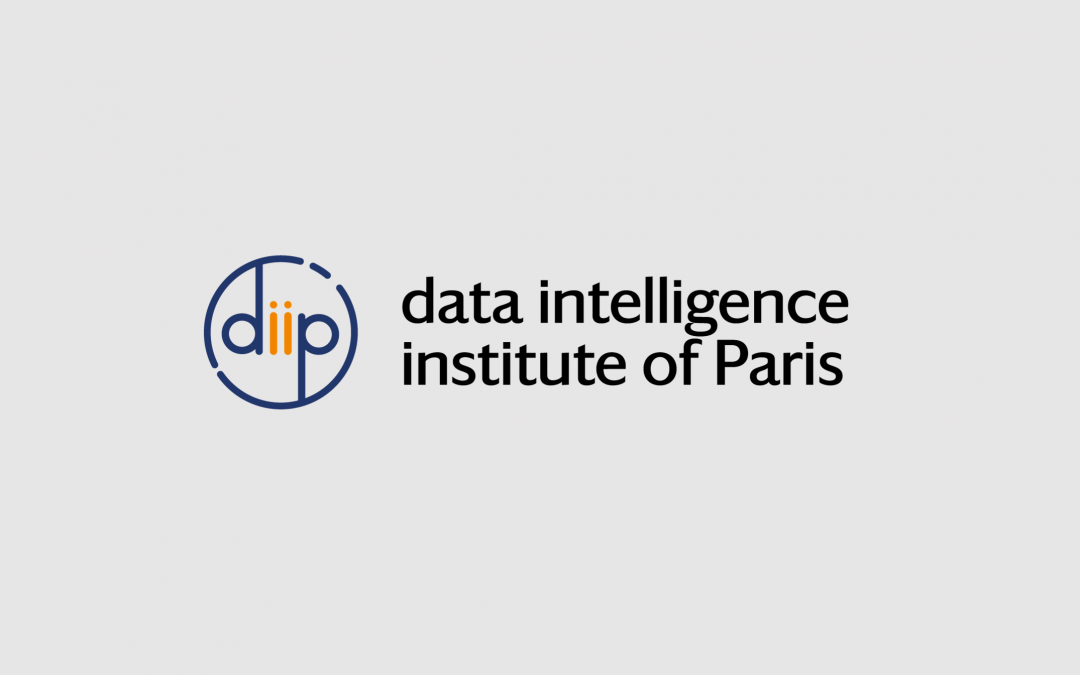
Diffusion Models Based Unpaired Image-to-Image Translation to Reveal Subtle Phenotypes
2023Masters Projects@Computer Science +Mathematics/Statistics+Biology+neurodevelopment #Image-to-image translation#Deep generative models#Diffusion models#Subtle Phenotypes#Neurodevelopment Project SummaryUnpaired image-to-image translation methods aim at learning a...
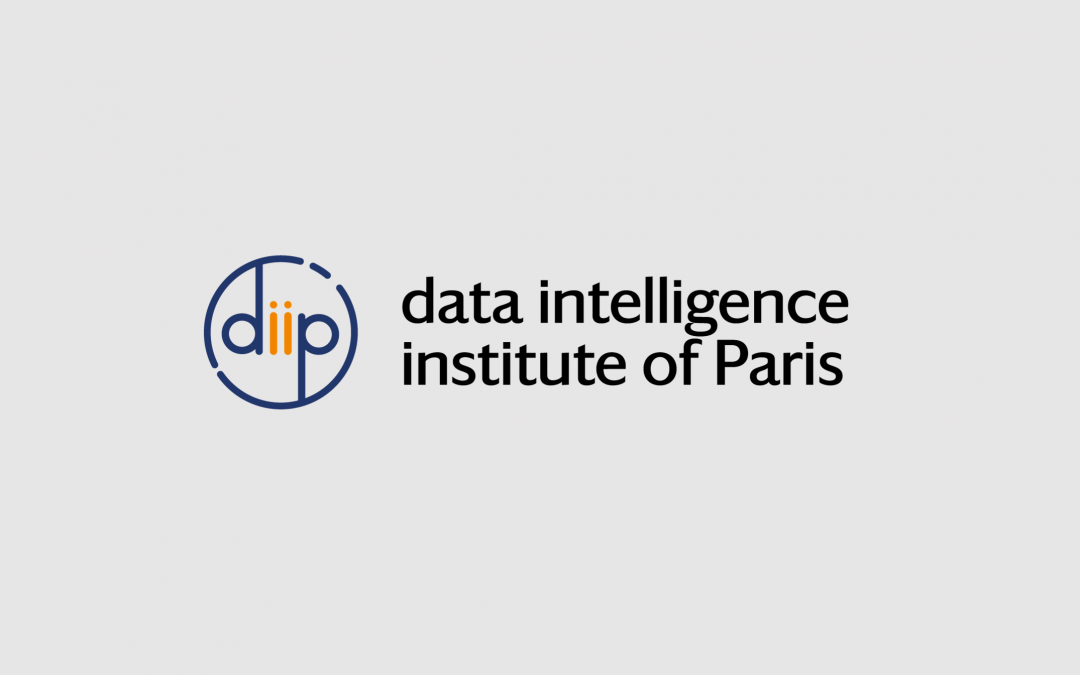
Generalization of a method enabling to update vineyard geographic databases from satellite data
2023Masters Projects@Computer Science +Earth Sciences/Geosciences #image time series analysis#deep learning#optical satellite imagery#agriculture monitoring#crop type mapping#vineyard#VENUS images Project Summaryto be updated. Camille Kurtz Projects in the same...