2021
PhD/ DIAI Projects
@Informatique, télécommunications et électronique de Paris (EDITE)
PhD student
Basile ROUSSE
(LIPADE, UPC)
Supervisors
Valérie GOLAZ (INED)
Sylvain LOBRY (LIPADE-EDITE, UPC)
Géraldine DUTHE (INED)
In this PhD, which stems from and strengthens an ongoing collaboration between LIPADE and INED, the candidate will develop deep learning-based methodologies using remote sensing data to predict indicators of the environment and environmental change for demographic analysis. As such, the objective of this topic is twofold: to propose methodological contributions for the large-scale extraction of environmental indicators and to analyze their contribution to spatial population and health analyses. How do these indicators compare with the existing environmental data? What results do they yield regarding the impact of environmental characteristics and environmental change on population structure and health in Sub-Saharan Africa?
We expect prime results in computer science (innovative methodologies) and demography (a better understanding of local inequalities in terms of population structure and health) as well as a contribution to fine remote sensing data analysis for population studies.
Context and summary
In a globalized context increasingly impacted by climate change, undergoing rapid population growth and urbanization, demographic studies would gain from considering fine-grained environmental data into account at the local, national, and transnational levels. However, this is not always possible in Sub-Saharan Africa, as matching harmonized demographic and environmental data are seldom available. The large amount of spatial data regularly acquired since 2015 (in 2019 only, Sentinel satellites from the European Space Agency produced 7.54 PiB of open-access data1) is an opportunity to produce standardized and up-to-date indicators. Several indicators have been developed to help understand geographical realities in a consistent (i.e., not location-dependent) manner. Among them, Local Climate Zones (LCZ) have been introduced by Stewart & Oke (2012) to label urban areas systematically. This classification system is based on geometrical and physical properties which makes it culture-independent. WUDAPT (World Urban Database and Access Portal Tools) provides an open-access map of the world following this legend that researchers can later use for a wide range of studies. This data has been used to understand energy usage, climate or geoscience modeling, or land consumption. An important amount of work has been dedicated in recent years to automatically generating such data from sensors such as Landsat 8 or Sentinel 2. In a research competition organized by the IEEE IADF, several methods have been proposed to map LCZ from Landsat, Sentinel 2, and OpenStreetMap data. Another recent study focused on the usage of Convolutional Neural Networks (CNNs) to tackle the task of automatically mapping LCZ using deep learning, and a large-scale benchmark dataset was proposed with a baseline of an attention-based CNN.
However, these works mostly focused on developed urban areas. For instance, the challenge targeted Berlin, Hong Kong, Paris, Rome, São Paulo, Amsterdam, Chicago, Madrid, and Xi’An. This is problematic, as developed cities are generally well-mapped through governmental censuses, and the spatial generalization of machine learning-based methods is challenging. Therefore, It is necessary to develop adapted methods for the global South, particularly sub-Saharan Africa. This PhD aims to develop domain adaptation methods using deep learning to map LCZ in this region accurately. These maps should comply with demographic constraints that are both spatial (the model should consider local climate characteristics) and temporal (the model should be able to map the region of interest during the period). Two studies have been carried out: mortality at the local scale in Antananarivo and malaria at the national scale in Burkina Faso through DHS surveys. In DHS surveys, a geospatial covariate dataset corresponding to the approximate locations of the clusters interviewed can be matched to household, male, and female datasets. The geospatial data stems from international programs aiming at providing estimates of environmental variables at the scale of planet Earth, such as population (Worldpop), temperature and rainfall (CRU, Worldclim), vegetation (VIP), urbanization (GHSL), . . . . Most of these are based on large scale estimates derived from Landsat data and are defined over a 10km buffer zone in rural areas, 2 km in urban areas.
However, a smaller buffer around a precise location has produced better results. We, therefore, can expect that a high-quality localized indicator such as LCZ in African urban metropolises would bring out better results when introduced in demographic analyses. This PhD falls beyond remote sensing, computer science, and demography. Many countries in sub-Saharan Africa experiences high climatic events but also strong population phenomena as rapid urbanization and migration. However, It is not always possible to have up to date population data in sub Saharan Africa to study these phenomena, which takes authorities away from taking the best decisions to manage these phenomena.
This PhD aims to accurately map the environment of sub Saharan countries through deep learning methods to study the effect of the environment, when precisely characterized, into population data. Two projects have been carried out linking the environment, described by local climate zones, to study mortality in Antananarivo at the local scale and malaria in Burkina Faso at the national scale.
For each project, domain adaptation method have been developed to generate a LCZ map from whichi households environment have been characterized. The characterization is then include into a multivariate analysis together whith the socio economic profile of the population to conclude on the effect of the environment in this phenomemna. In both project, the environment have proven to be significant in explaining the population data even when considering the socio economic profile.
Other projects
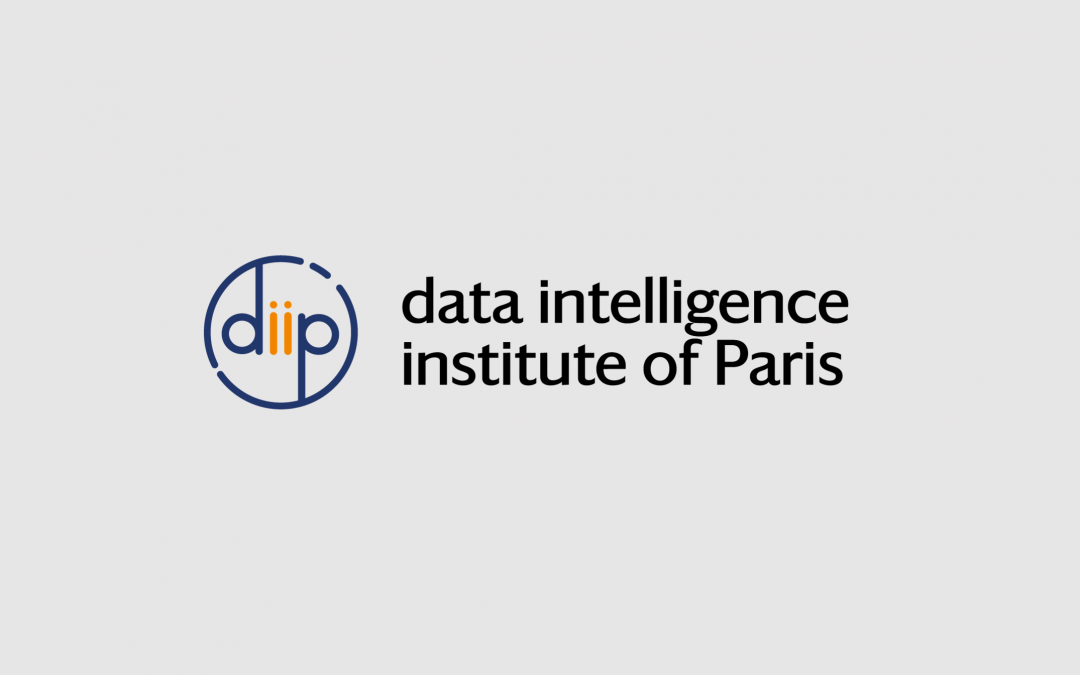
Deeply Learning from Neutrino Interactions with the KM3NeT neutrino telescope
2022 PhD/ DIAI Projects @AstronomyParticle physics and graph neural networks Santiago PENA MARTINEZ Project Summary A new generation of neutrino experiments is in the horizon looking to explore many of the open questions on neutrino properties and searching for...
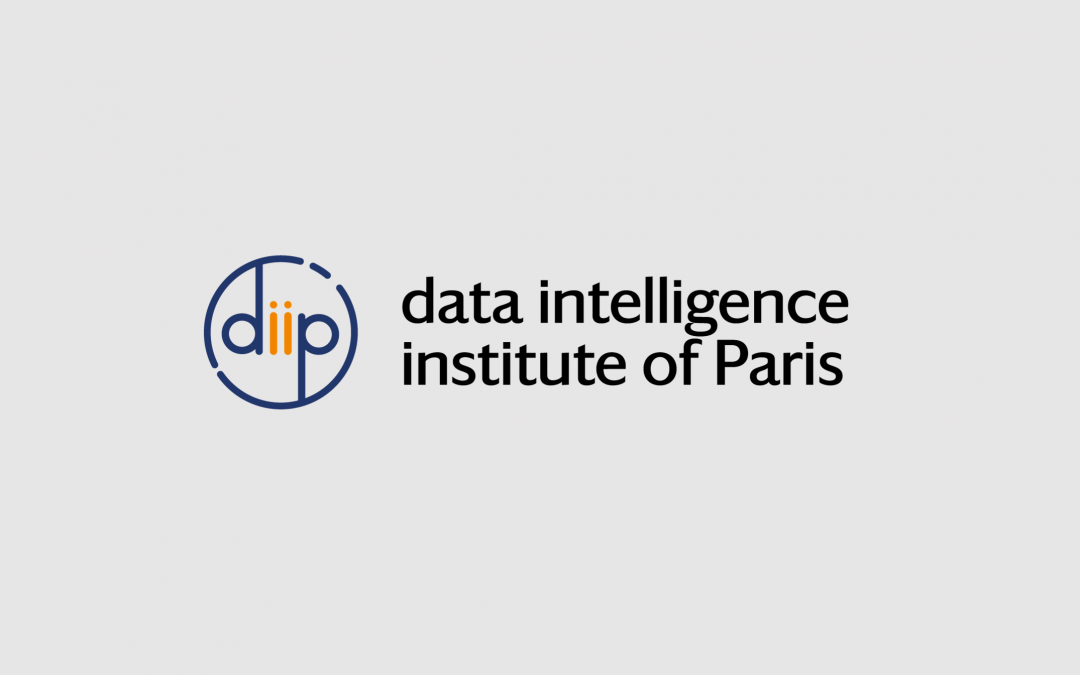
Metamorphoses and optimal transport for the multimodal registration of brain tumor images
2021 PhD/ DIAI Projects @ED386PhD studentGuillaume Serieys (MAP5, Université Paris Cité, CNRS) SupervisorJoan Alexis Glaunès Project Summary Medical imaging often involves nonlinear space-valued images, where pixel or voxel values belong to more complex...
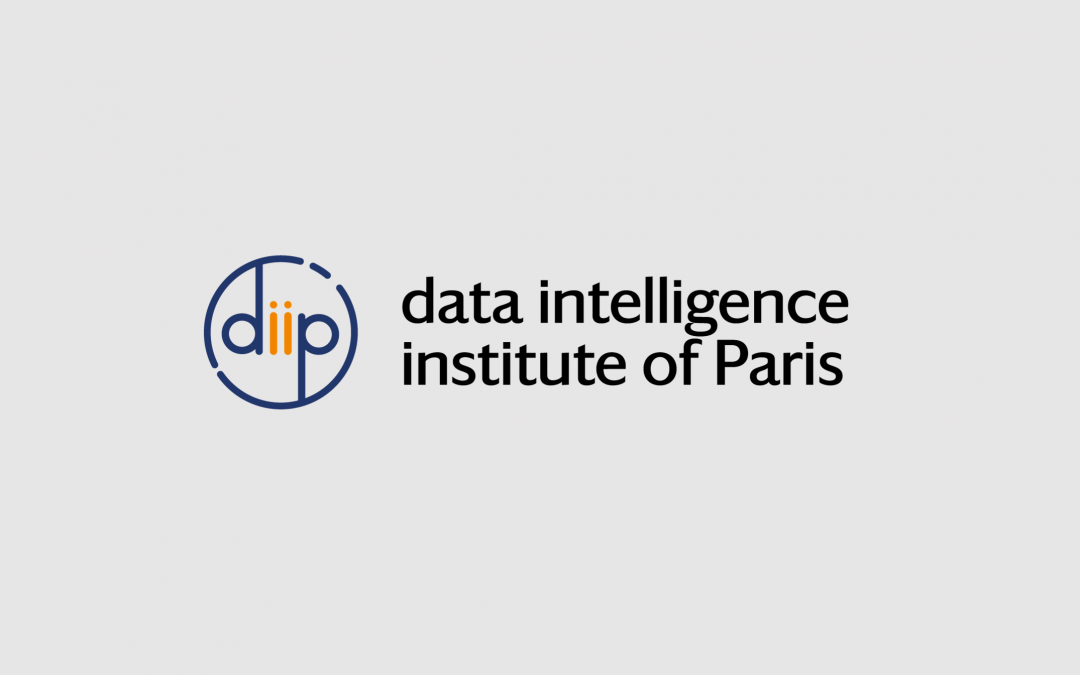
Learning the magneto-ionic side of the turbulence in the interstellar medium in radio-astronomy
2022 PhD/DIAI Projects @AstrophysiqueJack Berat(LPENS, UPC)Project Summary The phase transition from warm neutral medium to cold neutral medium in the interstellar medium (ISM) is affected by the magnetic field. The polarization of the synchrotron emission is one of...
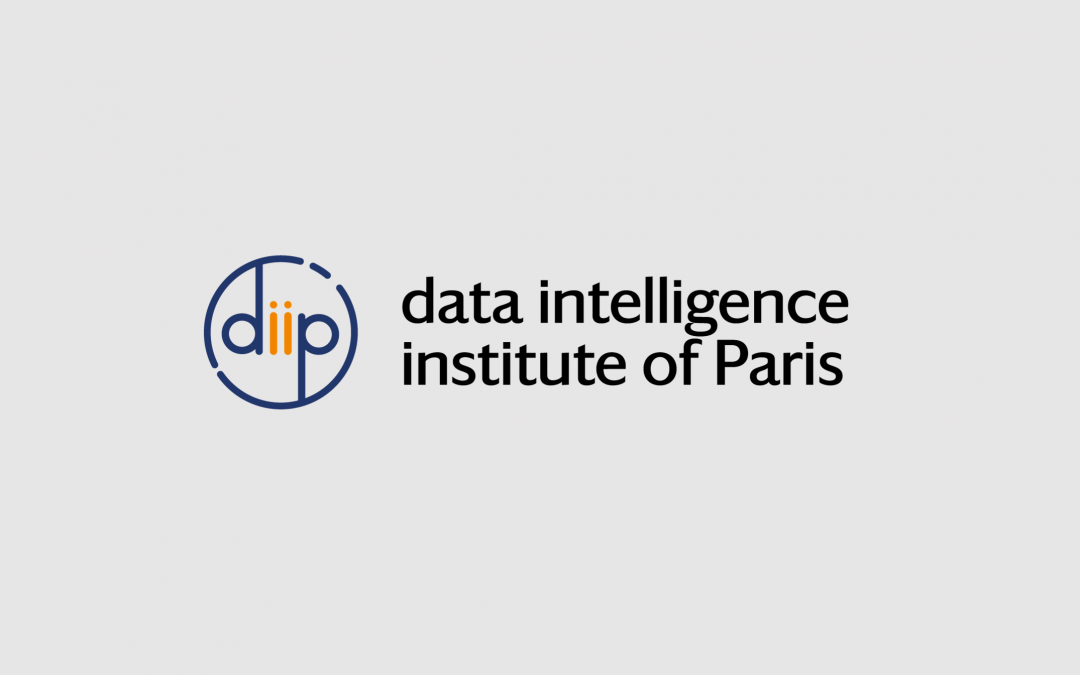
Leveraging multivariate geophysical and geochemical time series for monitoring volcanic systems: can we use machine learning?
2022 PhD/ DIAI Projects @Matthieu NougaretPhD student at Institut de Physique du Globe de Paris. Doctoral school 560.diiP, IdEx Université Paris Cité, ANR-18-IDEX-0001. Charles Le LosqUniversité de Paris, Institut de physique du globe de Paris, CNRSLise...