2024
Strategic Projects
@Chemistry
#simulated bodies
#amortised inferences
#inductive bias
#fetus
#MRI
Project Summary
Air pollution is the biggest environmental risk to health, which highlights the need for effective estimation of pollutant emissions. Improvements in satellite remote sensing technologies appear to be a game changer to better estimate pollutant emissions, yet it remains challenging to exploit the large amount of remote sensing data at high resolution. In this project, we draw on advanced deep learning techniques to address this challenge. With a focus on estimating nitrogen oxides (NOx) emissions from nitrogen dioxide (NO2) columns over East China. We first develop a data-driven deep neural network to emulate the state-of-the-art CHIMERE chemistry-transport model. We then embed it in a Physics-Informed Neural Network (PINN) for NOx emission estimation. We also utilize unsupervised domain adaptation techniques to handle the domain shift between varying data sources. The developed deep model will be applied to real NO2 data for 2019 to evaluate the impact of emission mitigation and for 2020 to evaluate the impact of COVID-19 on Chinese emissions.
Gaëlle Dufour
gaelle.dufour@lisa.ipsl.fr
- CNRS Senior Scientist at LISA (Laboratoire Interuniversitaire des Systèmes Atmosphériques UMR 7583)
Shen Liang
edwardliang11@gmail.com
Sylvain Lobry
Adriana Coman
Maxim Eremenko
Other projects
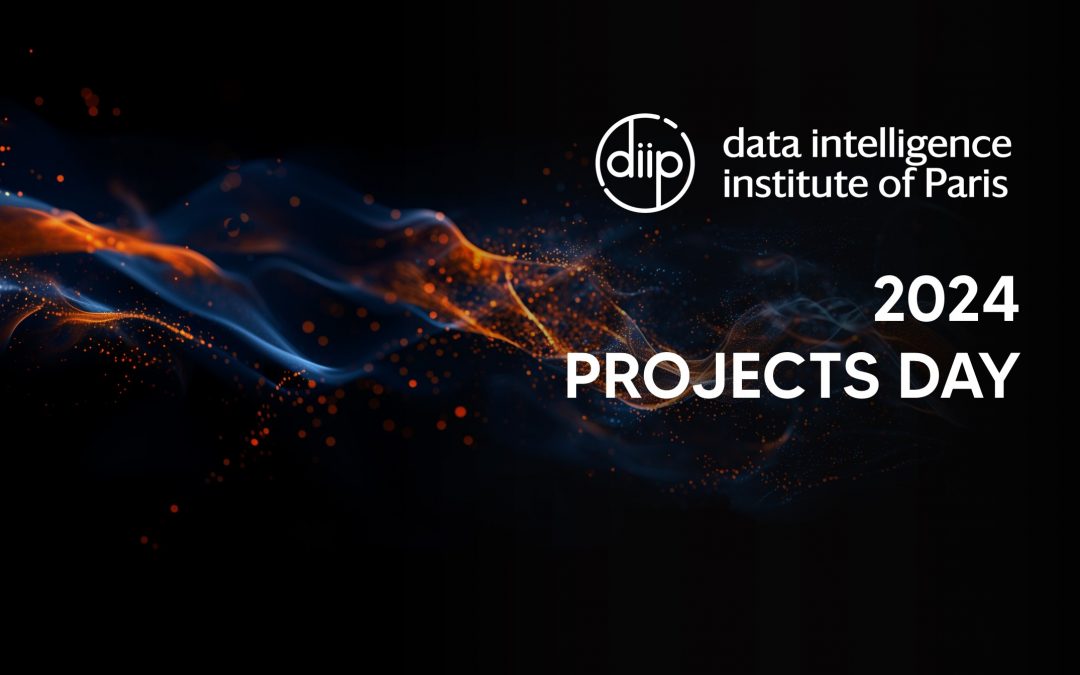
2024 diiP Projects Day
Date: Wednesday, December 4th Time: 9 AM to 5:30 PM Location: Vulpian Amphitheater, 12 rue de l’École de Médecine (75006 Paris) *Registration closes on November 11th *Free to attend This event brings together diiP researchers of 2024 to...
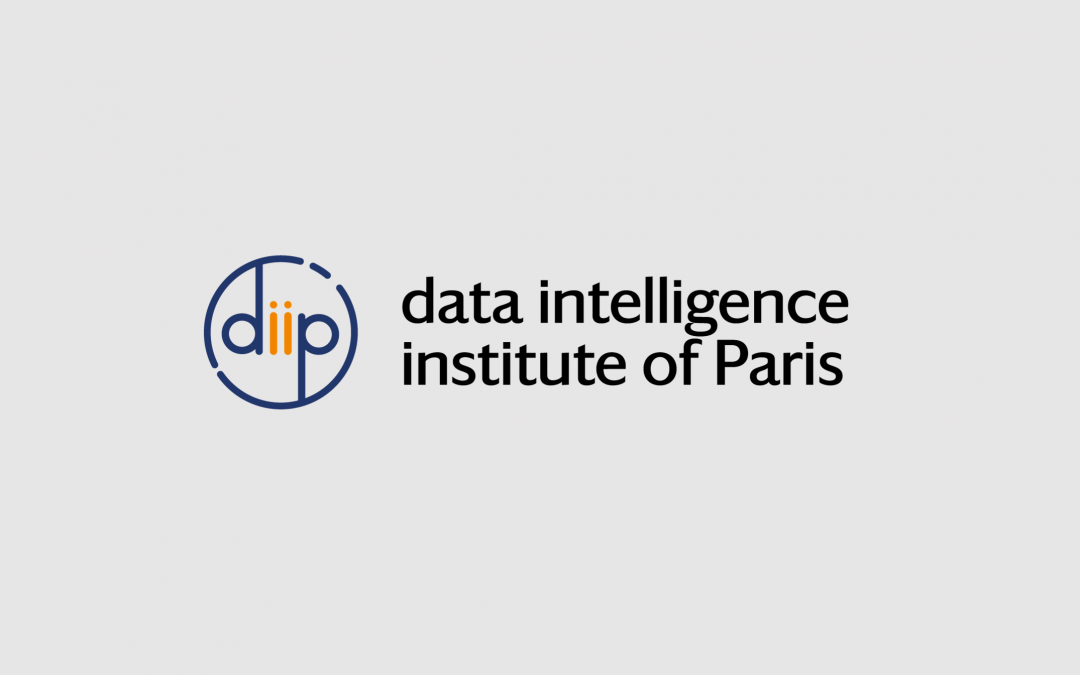
Deep Learning-based EEG Epilepsy Detection and Analysis
2023Strategic Projects@Computer Science +Medicine # EEG analysis#epileptic pattern detection#deep learning#active learning Project SummaryElectroencephalogram (EEG) is one of the most common and essential medical signal collected by neural scientists for the analysis...
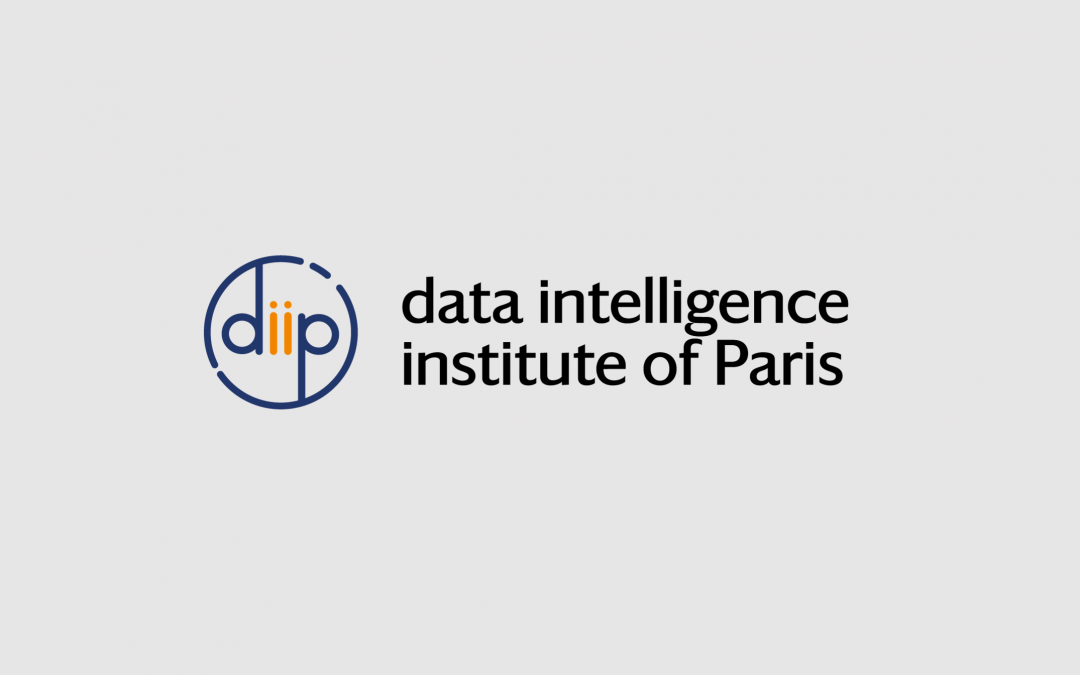
Semantic coherence integration for optimizing imaging retrieval systems in radiology
2023Strategic Projects@Computer Science +Medicine #medical imaging#computer vision#content-based image retrieval#deep learning#feature extraction#metric or ranking learning Project Summaryto be updated. Florence Cloppet Projects in the same discipline
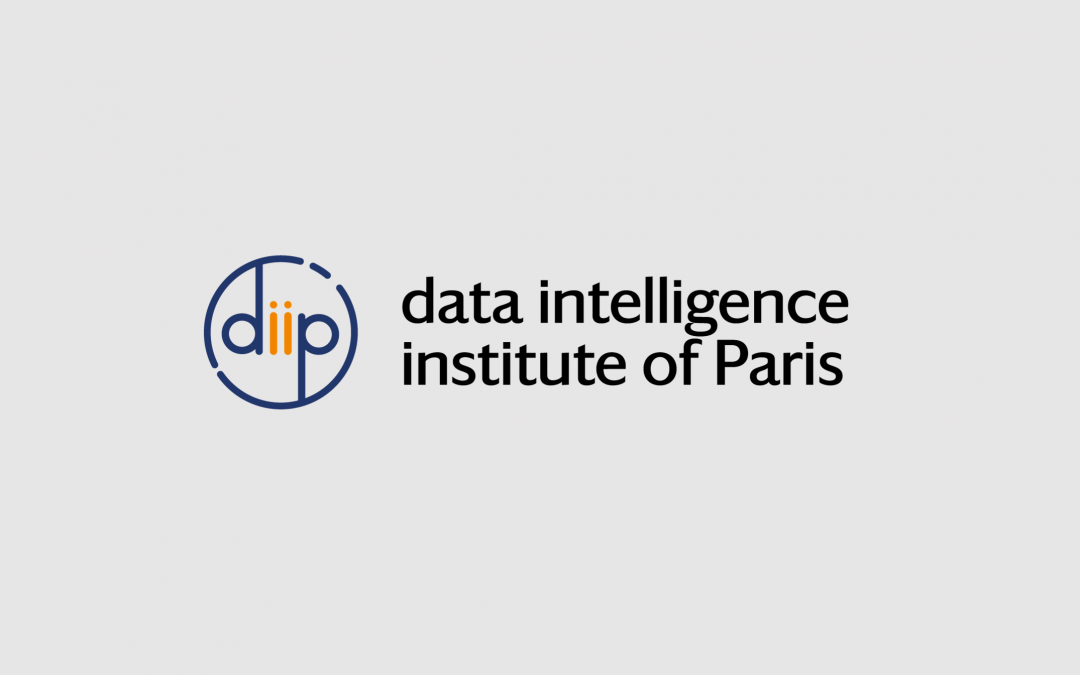
Multimodal assessment of the depth of sedation of severely ill patients in intensive care unit
2023Strategic Projects@Computer Science +Mathematics/Statistics+Engineering+Medicine+Neuroscience #anesthesia#signal processing#symbolic representations#physiological signals Project Summaryto be updated. Laurent Oudre Projects in the same discipline