Shen Liang
October 19, 4 PM (Central Eastern Time)
online (zoom)
Dr Shen Liang
(Université Paris Cité, diiP)
Shen Liang is a research associate at the Data Intelligence Institute of Paris (diiP) and affiliated with the Université Paris Cité. He has worked on a variety of data management and mining problems including time series analysis, semi-supervised learning, knowledge-guided deep learning and GPU-accelerated computation within various fields such as healthcare, manufacturing, geosciences and astrophysics. He holds a PhD in software engineering from Fudan University, China.
Abstract
In real-world applications, deep learning models are often faced with challenges from multi-source data with heterogeneous features. For example, in biomedicine, electrocardiography (ECG) signals of different patients can differ drastically even if they suffer from the same heart condition, thus a computer-aided diagnosis model that works well for one patient may work poorly for another; in astrophysics, simulation is widely used for neutrino event reconstruction, yet the distribution of simulated data often fails to align with that of real data, thus an event reconstruction model trained on simulated data may not be trustworthy on real data. Two effective solutions to the problem with multi-source data are domain adaptation and domain generalization. Domain adaptation attempts to transfer a model trained on one or multiple data sources to a data source where some data is already available, while domain generalization attempts to generalize a model training on multiple data sources to unknown future data. In this seminar, I will introduce some of the most commonly used methodologies for domain adaptation and generalization, and provide suggestions on when to and when not to apply these techniques in the face of multi-source data. Note that this seminar requires the audience to have basic knowledge on transfer learning and multi-task learning, which can be found in the seminar on June 18th.
Other seminars
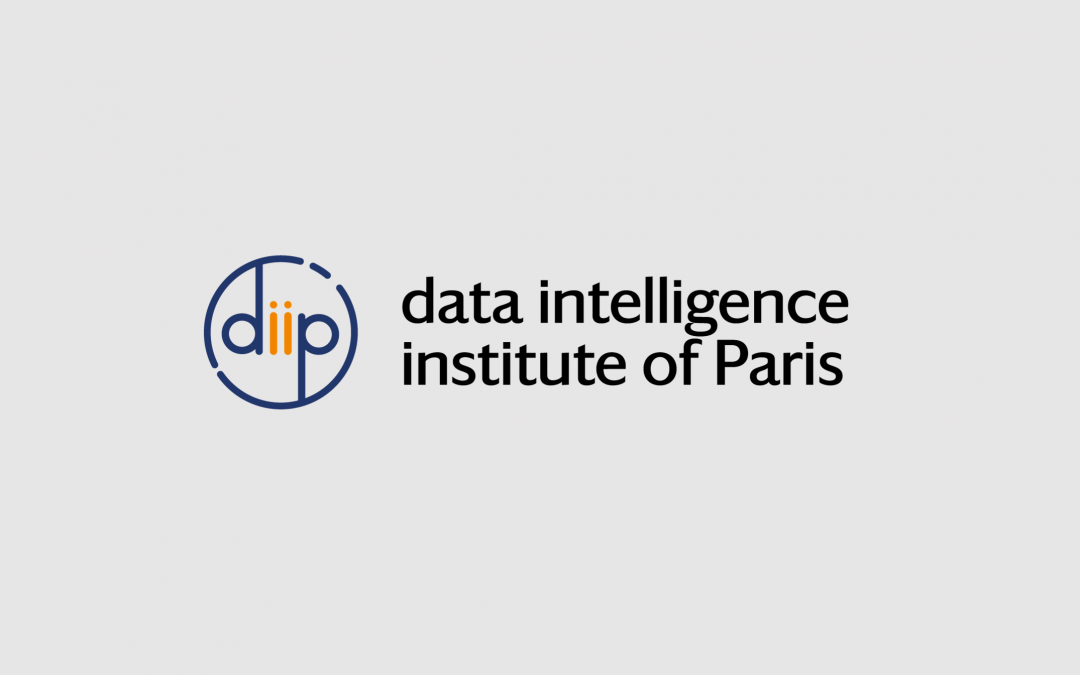
Shen Liang – Knowledge-guided Data Science
Shen LiangMay 18, 4 PM online (zoom) linkedinAbstract This tutorial presents an overview of knowledge-guided data science, a rising methodology in machine learning which fuses data with domain knowledge. We will present numerous case studies on this methodology...
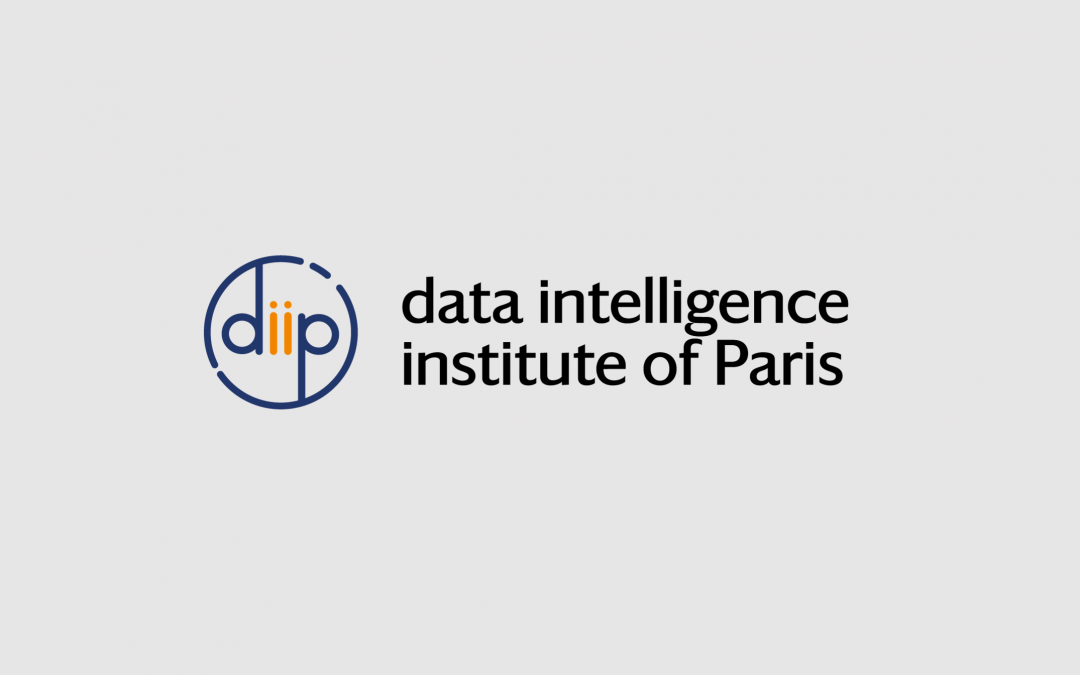
Shen Liang – Deep Transfer Learning and Multi-task Learning
Shen LiangJune 15, 4 PMonline (zoom) linkedinAbstract This tutorial provides an overview of two important and correlated (in many cases intersectional) topics in deep learning: transfer learning, and multi-task learning. Transfer learning focuses on...
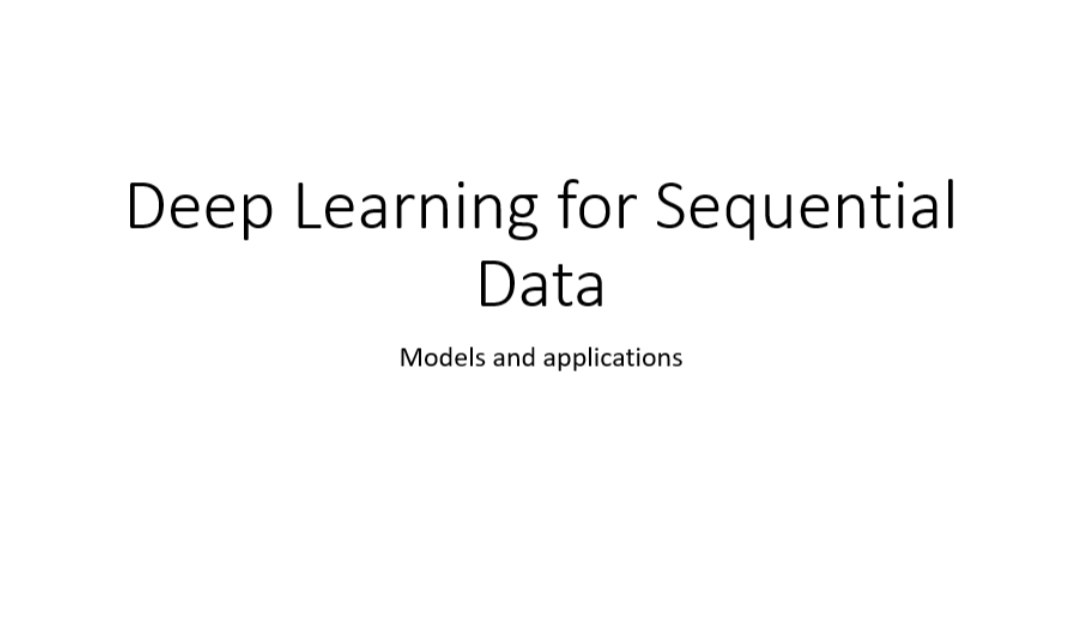
Foula Vagena – Deep Learning for Sequential Data: Models and Applications
Foula VagenaApril 13, 4 PMonline (zoom) Abstract Recurrent neural networks (RNNs) are a family of specialized neural networks for processing sequential data. They can scale to much longer sequences than would be practical for networks without sequence-based...
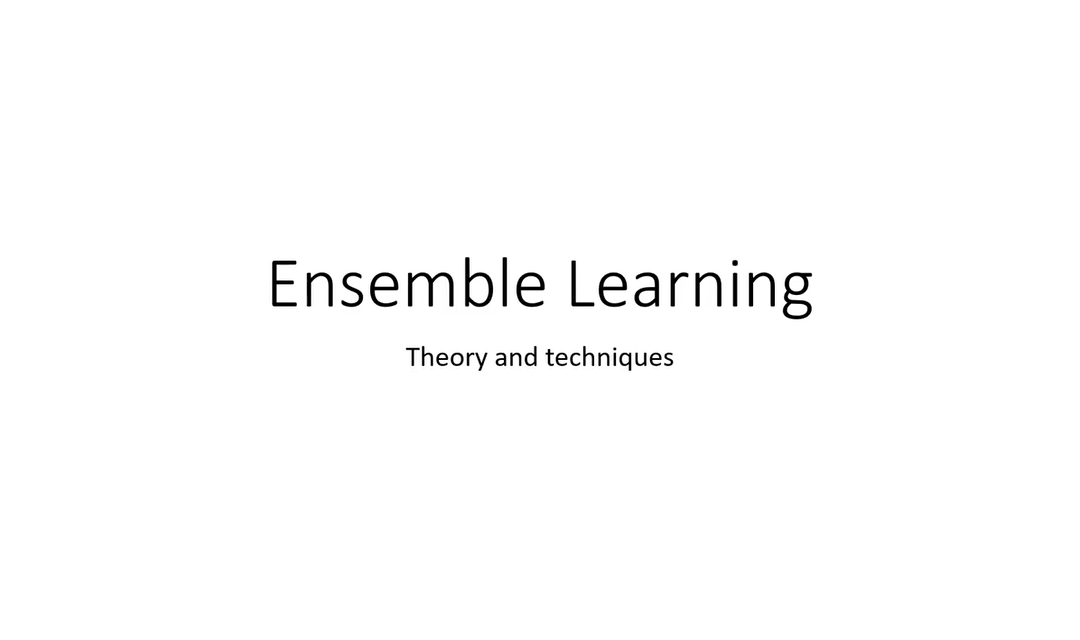
Foula Vagena – Ensemble Learning: Theory and Techniques
Foula VagenaDecember 15, 4pmonline (zoom) Abstract Ensemble learning is the process by which multiple models, such as classifiers or experts, are combined to solve a particular computational intelligence problem. Ensemble learning is primarily used to improve...