DIAI scholarships funded in 2021
The diiP is supporting scholarships related Data Intensive Artificial Intelligence (DIAI). Find out more below about the 6 selected PhDs in 2021.
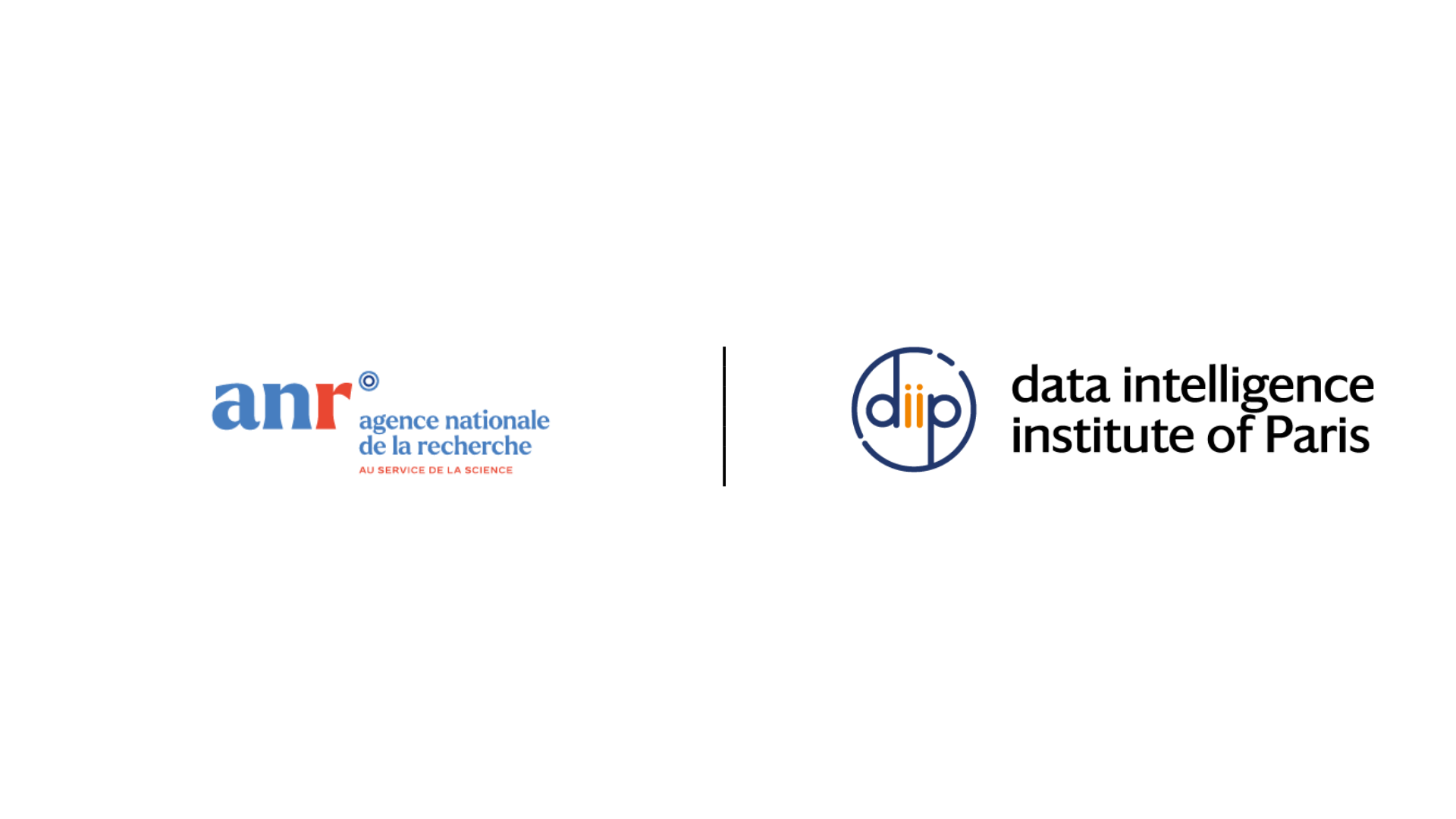
Presentation of the DIAI project
Extracting knowledge from the data means that we need to perform analysis tasks that are becoming increasingly complex as the amount of data, the number of observed variables, and the levels of noise (e.g., when measuring weak signals) in the measurements grow. Therefore, we are in need of novel methods that can cope with the scale of data and complexity of tasks that we face across applications in different domains. In this context, we need to develop new techniques that will advance the state of the art in the areas of data analytics, data science, and data intelligence (including artificial intelligence, machine learning, deep learning).
Scope of scholarships
These scholarships, which are proposed in the context of the 2019 Data Intensive Artificial Intelligence (DIAI) project that is co-funded by ANR, will support 12 PhD students (6 starting in 2021, and 6 in 2022), working on topics related to the intersection of data management/data analytics and machine learning/artificial intelligence, in order to address fundamental interdisciplinary challenges related to data analysis in modern science, industry, and society. Find out more below about the 6 selected PhD students in 2021.
ED 127 : Astronomie & Astrophysique
Cosmologie – amas de galaxies – intelligence artificielle
PhD student: Nicolas CERARDI (AIM, UPC)
Supervisor: Marguerite PIERRE (AIM, UPC)
La thèse a pour cadre le projet international de cosmologie XMM-XXL. Le but est de déterminer de manière indépendante l’équation d’état de l’Energie Sombre en utilisant des observations d’amas de galaxies en rayons X. Les observations sont obtenues à l’aide du satellite XMM de l’Agence Spatiale Européenne.
Le principe repose sur le fait que le nombre d’amas de galaxies formés au cours du temps dépend de manière critique de certains paramètres cosmologiques comme la densité de matière dans l’univers et le taux d’accélération de l’expansion (dont la découverte valut le prix Nobel à ses auteurs en 2011). Les observations en rayons X nous renseignent de manière directe sur l’existence, la masse et la distance des amas de galaxies.
Des résultats préliminaires portant sur un échantillon partiel ont été obtenus en 2018 ; ils ont fait l’objet de plusieurs communiqués de presse et ont été publiés dans un numéro spécial d’Astronomy and Astrophysics. La thèse proposée se situe dans la dernière phase du projet XXL et portera sur l’échantillon complet d’amas.
L’analyse cosmologique utilise le principe actuel du ‘forward modelling’ c’est à dire que les données XMM utilisées dans cette étude sont des quantités directement observables telles que le flux, la couleur, la taille apparente et le redshift des amas de galaxies détectés par XXL. Cette méthode permet de s’affranchir du fastidieux calcul direct de la masse des amas (qui dépend de la cosmologie).
Pour cette thèse, les méthodes d’analyse traditionnelles des données (type MCMC, utilisé jusqu’à présent par le projet) seront remplacées par une approche novatrice de type Intelligence Artificielle (précisément: Inference Free Likelihood + Approximate Bayesian Computation). Ceci permet d’améliorer et d’accélérer considérablement l’analyse cosmologique et, en même temps, d’explorer d’éventuelles dégénérescences entre les paramètres cosmologiques et ceux décrivant l’évolution de la physique des amas. L’idée de base est de considérer ces derniers comme des paramètres de nuisance qui se combinent aux autres incertitudes comme le bruit stochastique sur le nombre d’amas (dû à la taille finie du relevé) et les incertitudes sur la mesure des quantités X. Un des principaux problèmes pratiques à résoudre sera donc l’optimisation des simulations dans un espace à 11 dimensions, pour entrainer le réseau.
Il s’agira de:
(1) Finaliser le catalogue d’amas en vue de l’analyse cosmologique
(2) Optimiser la structure et le mode d’entrainement du réseau de neurones en fonction des contraintes observationnelles; modéliser les erreurs statistiques et les erreurs systématiques à l’aide de simulations
(3) Appliquer le réseau à l’analyse cosmologique du catalogue final d’amas XXL.
(4) Prédire les performances de la méthode pour les satellites X eROSITA (2019) et Athena (2032).
Les logiciels de base sont disponibles (cosmologie, réponse des instruments d’XMM, programmes IMNN+ABC) ainsi que les codes permettant de créer les simulations nécessaires à l’entrainement du réseau de neurones.
La bibliothèque de programmes pour l‘apprentissage profond est en langage python et a été adaptée aux spécificités du projet XXL. Les calculs sont effectués à distance au Centre de Calcul de l’IN2P3 à Lyon.
La thèse aura lieu dans le cadre très dynamique de l’équipe de Saclay (maître du projet XXL) et du consortium international XXL, regroupant directeurs de recherche, chercheurs, post-doc et étudiants.
Site du projet XXL.
ED 130 : Informatique, télécommunications et électronique de Paris (EDITE)
Exploration intelligente de lames histologiques.
PhD student: Zhuxian GUO (LIPADE, UPC)
Supervisors: Nicolas LOMÉNIE (LIPADE, UPC), Camille KURTZ (LIPADE, UPC), Henning MÜLLER (unité Health Sierre, Suisse)
The rapidly emerging field of computational pathology needs new paradigms and tools coming from computer sciences in the broadest sense of the science. Our team is developing many projects in the field including setting up international challenges (like TissueNet : detect lesions in cervical biopsies hosted by French Society of Pathology and Health Data Hub in collaboration with Driven Data). The ultimate goal is to be able to provide objective diagnosis, therapeutic response prediction and identification of new morphological features of clinical relevance within the next decade. The team has a pending patent in the field and is part of a phase 2 clinical trial for immuno-therapy as a digital companion test (starting April 2021). We are involved in the community of the French Society of Pathology and will start a PRT-K project for translational clinical research in oncology.
In our team, we believe that the next step will rely on the integration of the computer vision/machine learning pipelines into the clinical setting. We already start to get a good deal of annotated WSI (gigapixel whole slide images ‘WSI’ for each patient/exam) by experts and collaborations with hospitals. We need to go the next step right now :
- as a data science playground, we need to explore deep learning architectures to perform seamless exploration of all the data annotated across various tissues and markers to propose a scaling pipeline of processing and sharing of data among researchers and clinicians;
- the integration with genomic data will also be of utmost importance in the coming decade either to validate theory in life sciences based on the observation of tumoral micro-environment or to predict genomic class based on phenotypic observation at the tissue level (publication submitted in our team in Journal of Hepathology);
- how to integrate these paradigms (for physicians) into the concept of the XX1st century microscope made possible with the advent of these new high-throughput high resolution scanners possibly with multiple markers.
Prediction of demographic indicators from remote sensing images
PhD student: Basile ROUSSE (LIPADE, UPC)
Supervisors: Valérie GOLAZ (INED), Sylvain LOBRY (LIPADE-EDITE, UPC), Géraldine DUTHE (INED)
In this PhD, which stems from and strenghtens an on-going collaboration between LIPADE and INED, the candidate will develop deep learning based methodologies using remote sensing data to predict indicators of the environment and environmental change, for demographic analysis. As such, the objective of this topic is twofold: to propose methodological contributions for the large-scale extraction of diachronic environmental indicators and to analyze their contribution to spatial population and health analyses. How do these indicators compare with the existing environmental data? What results do they yield in terms of the impact of environmental characteristics and environmental change on population structure and health in Sub-Saharan Africa?
We expect prime results in the field of computer science (innovative methodologies) and demography (a better understanding of local inequalities in terms of population structure and health) as well as a contribution to the use of fine remote sensing data analysis for population studies. Contexte and Subject In a globalized context increasingly impacted by climate change, undergoing rapid population growth and urbanization, demographic studies would gain to better take environmental data into account and be carried out at the transnational level. However, this is not always possible in Sub-Saharan Africa, as matching harmonized demographic and environmental data are seldom available. The large amount of spatial data regularly acquired since 2015 (in 2019 only, Sentinel satellites from the European Space Agency produced 7.54 PiB of open-access data1) are an opportunity to produce standardized and up-to-date indicators. Several indicators have been developed to help understanding geographical realities in a consistent (i.e. not location dependent) manner. Among them, local climate zones (LCZ) have been proposed by WUDAPT (World Urban Database and Access Portal Tools) to systematically label urban areas. Their goal is to provide a map of the world following this legend, in open-access, that can later be used by researchers for a wide range of studies. This data has been used to understand energy usage, climate or geoscience modeling or land consumption. An important amount of work has been dedicated in the recent years to the automatic generation of such data, from sensors such as Landsat 8 or Sentinel 2. In a research competition organized by the IEEE IADF, several methods have been proposed to map LCZ from Landsat, Sentinel 2 and OpenStreetMap data. Another recent study focused on the usage of Convolutional Neural Networks (CNNs) to tackle the task of automatically mapping LCZ using deep learning and a large scale benchmark dataset was proposed in, with a baseline of an attention-based CNN.
However, these works mostly focused on developed urban areas. For instance, the challenge of targeted Berlin, Hong Kong, Paris, Rome, São Paulo, Amsterdam, Chicago, Madrid, and Xi’An. This is problematic, as developed cities are generally well mapped through governmental censuses, and that spatial generalization of machine learning based methods is a challenge. It is therefore necessary to develop adapted methods for the global South. In DHS surveys, a geospatial covariate dataset corresponding to the approximate locations of the clusters interviewed can be matched to household, male and female datasets. The geospatial data stems from international programs aiming at providing estimates of environmental variables at the scale of planet Earth, such as population (Worldpop), temperature and rainfall (CRU, Worldclim), vegetation (VIP), urbanisation (GHSL), . . . . Most of these are based on large scale estimates derived from Landsat data and are defined over a 10km buffer zone in rural areas, 2 km in urban areas. However, a smaller buffer around a precise location has been proven to bring out better results . We therefore can expect that a high quality localised indicator such as LCZ in African urban metropolises would bring out better results when introduced in demographic analyses.
ED 560 : Sciences de la Terre et de l’Environnement et Physique de l’Univers de Paris (STEP'UP)
Dark energy studies with the Vera Rubin observatory LSST & Euclid-developing a combined cosmic shear analysis with bayesian neural networks
PhD student: Justine ZEGHAL (AstroParticule & Cosmologie, UPC)
Supervisors: Eric AUBOURG (APC, UPC), Alexandre BOUCAUD (APC, UPC), Cécile ROUCELLE (APC, UPC).
During the last decade, cosmology has entered a precision era, leading to the prevalence of the standard cosmological model, ΛCDM. Nevertheless, the main ingredient of this model, dark energy, remains mysterious while dominating the energy budget of the Universe. Its comprehension is the current Graal of this domain. The next generation of cosmological surveys, among which Legacy Survey of Space and Time (LSST) of the Vera Rubin Observatory (on the ground) & Euclid (in space), both starting their data taking in 2023, are in that regard the most important projects for the next 10 years.
These surveys, when combined, will map thousands of square degrees of sky in a multiwavelength manner with sub-arcsec resolution. This will result in the detection of several tens of billions of sources, enabling a wide range of astrophysical investigations and providing unprecedented constraints on the nature of dark energy and dark matter. The scope of the PhD topic is at the crossing of the two surveys. More precisely, the PhD topic discussed here is focused on developing analyses for weak gravitational lensing combining the data of LSST and Euclid.
If successful, the implications of this work could drastically reduce the bias on cosmic shear measurements and release an essential tool for observational cosmology to the community. Not to mention that LSST commissioning data will become available for science in 2023, during the PhD thesis, making these studies all the more interesting as the scientific environment will be extremely dynamic and competitive.
ED 386 : Sciences Mathématiques de Paris Centre
Machine Learning for Survival Data Prediction with applications to Primary Immunodeficiences data
PhD student: Ariane CWILLING (MAP5, UPC)
Supervisors: Olivier BOUAZIZ (Sciences Mathématiques de Paris Centre, UPC), Vittorio PERDUCA (MAP5, UPC)
This PhD project is at the frontier between mathematics, artificial intelligence and applications to health. Its aim is to develop new statistical and machine learning methods for survival analysis with a focus on the prediction of the time to event rather than on the traditional estimation of hazard rates. To palliate the opaqueness that characterises automatic learning algorithms, we will develop methods for interpretable machine learning adapted to our censored data context and we will study their properties to provide statistical guarantees. We plan to apply our methods to primary immunode ciencies (PIDs) patients data for which no prediction methods currently exist.
Query analytics in Cypher
PhD student: Alexandra ROGOVA (IRIF, DI ENS)
Supervisors: Amélie GHEERBRANT (IRIF, UPC), Leonid LIBKIN (Laboratory for Foundations of Computer Science, University of Edinburgh)
In this PhD topic proposal we will focus on the graph data management system Neo4j and its associated query language Cypher. Neo4j is currently the leader among graph database engines [2] and Cypher is now a widespread query language. We are more specifically interested in extending Cypher in order to mix database querying and analytics tasks. For example, we would like to be able to express queries finding shortest paths, counting triangles, computing PageRank, or running any other useful graph algorithm. Some of these algorithms can actually already be called in Cypher using built-in procedures (there is even a ”Graph Data Science” Neo4j plugin devoted to graph analytics [3]). For instance, we can easily modify our previous query to return the shortest path between ”SARS-CoV-1” and ”SARS-CoV-2” :
MATCH (r1: Virus { name : “SARS-CoV-2”})
MATCH (r2: Virus { name : “SARS-CoV-1”})
MATCH path = shortestpath (( r1 ) -[child_of*] -( r2 ))
RETURN path ;
However it is not yet possible to define from scratch in Cypher random graph algorithms of interest. We would like to study extensions of Cypher allowing this. When extracting data from a graph using traditional methods, one must constantly switch between query languages (to gather raw data) and analytics frameworks (to extract useful information from the raw data). In this context, merging the querying and analytics by enriching the query language (here Cypher) with analytic features is appealing. This is not yet a new trend in industry but it might very well become one : in graph database research, it just started picking up steam. For now work has been done exclusively on the RDF side, but ideas can be readily adapted to property graphs as both models effectively encode digraphs (think of the RDF triple as [node, edge label, node]).
À lire aussi
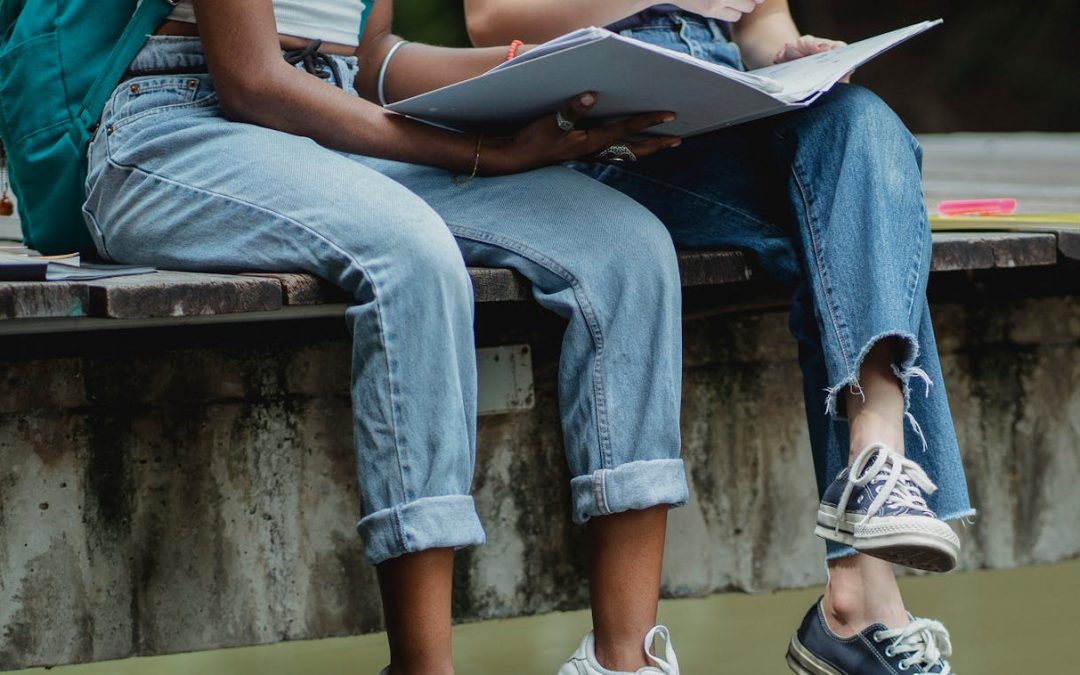
diiP Summer School: June 10-14, 2024
The diiP is organizing a Summer School on Data Science (with a focus on deep learning data analytics techniques), on Jun10-14. Read the details below, and register now! The first diiP Summer School on Data Science (dSDS) will be held...
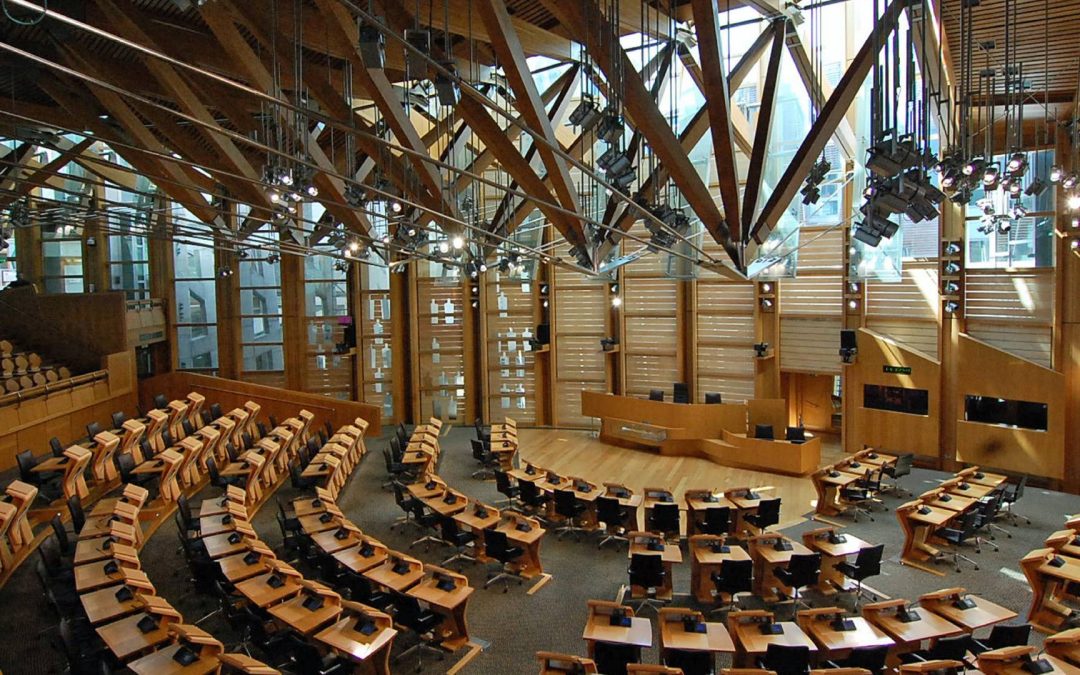
diiP Projects Day: December 6th, 2023
Join us for the diiP Projects Day, an in-person event that will highlight past and upcoming projects, offer opportunities for discussions and networking, and host Prof. Joseph Sifakis (Turing Award winner, 2007) for the last Distinguished Lecture of 2023....
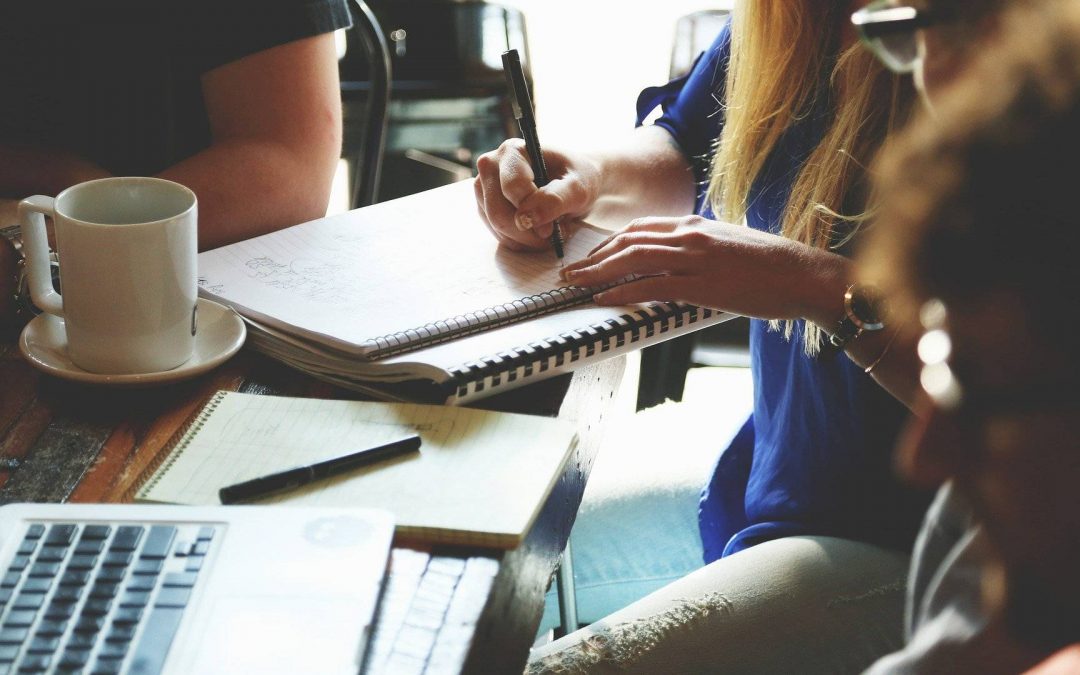
2024 internship opportunities for Master’s students
Below are listed internship opportunities currently offered by diiP. These offers are open to second year Master’s students. Supervised by: Nicolas Loménie (Université Paris Cité) Description: Digital Pathology is on the rise. Most...
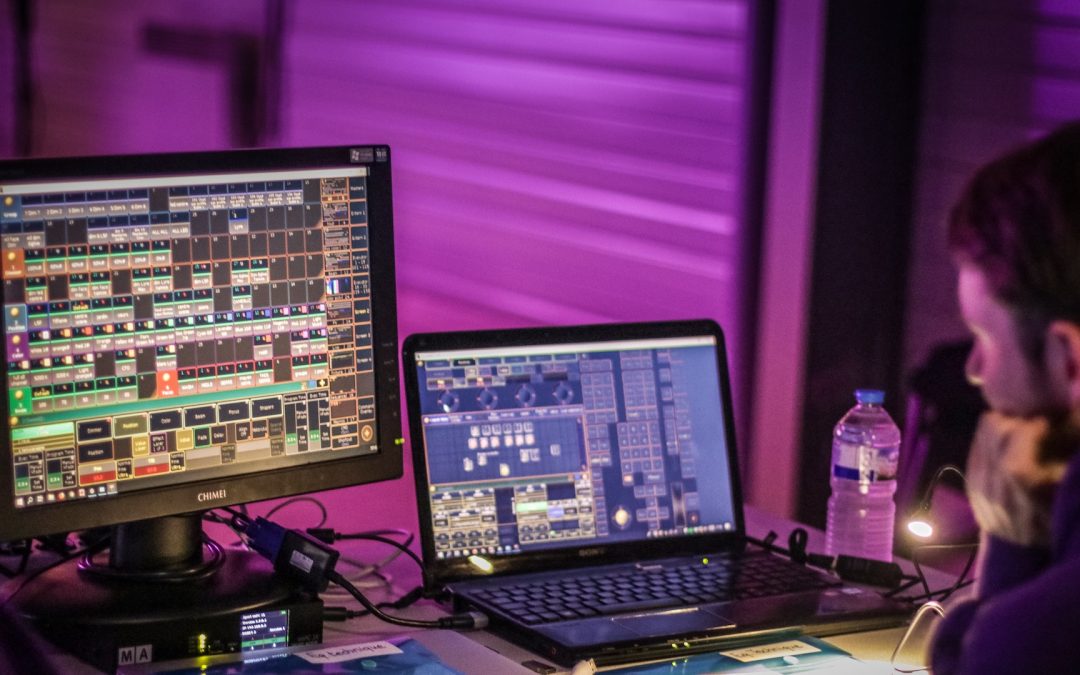
LRCS is looking for an AI Engineer for the PEPR Battery OpenStorm Project
The Laboratoire de Réactivité et Chimie des Solides is looking for an AI engineer for the PEPR Battery OpenStorm Project (France2030). The LCRS; located in Amiens, France, is looking for an AI engineer to help in the development of...